In today’s data-driven landscape, the power of predictive analytics has become a strategic necessity for organizations across industries. However, choosing the right predictive analytics tool can be a daunting task, given the multitude of options available in the market. In this article, we embark on a comprehensive journey to explore and compare some of the leading predictive analytics tools, shedding light on their features, strengths, and weaknesses. Join us as we navigate the terrain of data analysis and prediction.
The Significance of Predictive Analytics Tools
Predictive analytics tools are the engines that drive data-driven insights. They enable organizations to harness historical data and forecast future trends, making informed decisions that can shape the course of their business. As the demand for predictive analytics continues to soar, selecting the right tool becomes pivotal.
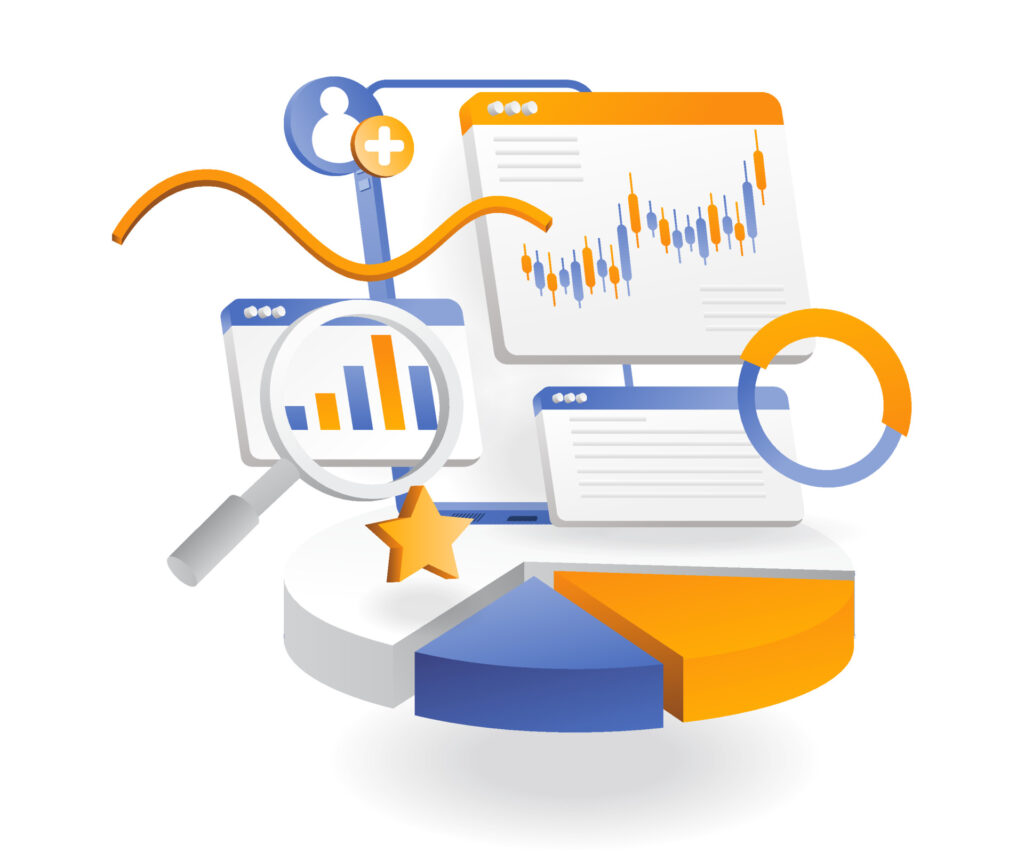
Predictive Analytics Tool Comparison
In our quest to unravel the predictive analytics landscape, we delve into a comparative analysis of some of the most prominent tools available today:
1. Python with scikit-learn:
- Strengths: Python’s versatility and scikit-learn’s rich library of machine learning algorithms make it a favourite among data scientists. It offers flexibility, extensive documentation, and a vibrant community.
- Weaknesses: While powerful, Python can be complex for beginners. Implementation may require additional coding skills.
2. R:
- Strengths: R is a statistical powerhouse, renowned for its statistical modeling capabilities. It excels in data visualization, and statistical analysis, and offers numerous packages for predictive modelling.
- Weaknesses: R can have a steeper learning curve compared to some other tools, and its data-handling capabilities may require optimization.
3. RapidMiner:
- Strengths: RapidMiner is known for its user-friendly interface and drag-and-drop functionality. It’s suitable for users with varying levels of technical expertise and offers a wide range of data preprocessing and modelling tools.
- Weaknesses: While user-friendly, some advanced features may require scripting. Licensing costs can also be a consideration for larger enterprises.
4. KNIME:
- Strengths: KNIME provides an open-source platform with strong data integration capabilities. Its modular approach allows users to build customized workflows and incorporate various machine-learning algorithms.
- Weaknesses: KNIME may require a bit more setup and configuration compared to other tools. Users with limited technical skills may find certain aspects challenging.
5. SAS Enterprise Miner:
- Strengths: SAS is renowned for its analytics expertise, and Enterprise Miner is no exception. It offers a comprehensive suite of tools for data preparation, modelling, and deployment. It’s highly regarded for its scalability and reliability.
- Weaknesses: SAS tools are often associated with higher costs. Smaller organizations may find it less budget-friendly.
6. IBM Watson Studio:
- Strengths: IBM Watson Studio combines powerful machine learning capabilities with IBM’s AI expertise. It offers a collaborative environment for data scientists and data engineers, making it suitable for enterprise-level projects.
- Weaknesses: Like SAS, IBM Watson Studio can be on the higher end of the pricing spectrum. It’s a better fit for organizations with substantial analytics needs.
Conclusion
The choice of a predictive analytics tool is a critical decision that can significantly impact an organization’s ability to extract actionable insights from data. Each tool we explored has its strengths and weaknesses, making it essential to align your choice with your specific needs, technical resources, and budget constraints.
Whether you opt for the flexibility of Python, the statistical rigour of R, the user-friendliness of RapidMiner, the modularity of KNIME, the comprehensive capabilities of SAS, or the AI prowess of IBM Watson Studio, the key lies in leveraging the tool’s strengths to empower your predictive analytics journey.
As technology evolves and data analytics advances, the landscape of predictive analytics tools will continue to evolve. Therefore, periodic reassessment of your toolset is recommended to ensure your organization remains at the forefront of data-driven decision-making.
Stay tuned for more insights on predictive analytics and data-driven strategies as we continue to explore the ever-evolving world of data analytics.